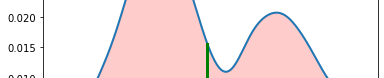
Chebyshev's Inequality
LearnDataSci is reader-supported. When you purchase through links on our site, earned commissions help support our team of writers, researchers, and designers at no extra cost to you.
What is Chebyshev's Inequality
Chebyshev's inequality is a theory describing the maximum number of extreme values in a probability distribution. It states that no more than a certain percentage of values ($1/k^2$) will be beyond a given distance ($k$ standard deviations) from the distributionβs average. The theorem is particularly useful because it can be applied to any probability distribution, even ones that violate normality, so long as the mean and variance are known. The equation is:
$$ Pr(|X-\mu| \geq k \times \sigma) \leq \frac{1}{k^2} $$
In plain English, the formula says that the probability of a value (X) being more than k standard deviations (π) away from the mean (π) is less than or equal to $1/k^2$. In that formula, $k$ represents a distance away from the mean expressed in standard deviation units, where $k$ can take any above-zero value.
As an example, let's calculate the probability for $k=2$.
$$ Pr(|X-\mu | \geq k) \leq \frac{\sigma^2}{k^2} $$
Although, the inequality is valid for any real number $k>0$, only the case $k>1$ is practically useful.
As an example, let's calculate the probability for k=2.
$$ Pr(|X-\mu|\geq 2 \times \sigma) \leq \frac{1}{4} $$
The answer is that no more than ΒΌ of values will be more than 2 standard deviations away from the distributionβs mean. Note that this is a loose upper bound, but it is valid for any distribution.
Finding the reverse: Number of values within a range
Chebyshevβs inequality can also be used to find the reverse information. Knowing the percentage of values outside a given range also by definition communicates the percentage of values inside that range. $\text{Percentage inside} = 1 - \text{Percentage outside}$. In other words, the maximum number of values within $k$ standard deviations of the mean will be $1 β 1/k^2$.
In the previous example, we calculated that no more $1/4$ of values will be more than 2 standard deviations away from the distributionβs mean. To find the reverse, we calculate $1 - 1/k^2 = 1 - 1/4 = 3/4$. In other words, no more than $3/4$ of values will be within 2 standard deviations from the distributionβs mean.
Applications
Chebyshev's inequality theorem is one of many (e.g., Markovβs inequality theorem) helping to describe the characteristics of probability distributions. The theorems are useful in detecting outliers and in clustering data into groups.
A Numerical Example
Suppose a fair coin is tossed 50 times. The bound on the probability that the number of heads will be greater than 35 or less than 15 can be found using Chebyshev's Inequality.
Let $X$ be the number of heads. Because $X$ has a binomial distribution:
- The expected value will be: $ \mu = n \times p = 50 \times 0.5 = 25$
- The variance will be: $ \sigma^2 = n \times p \times (1-p) = 50 \times 0.5 \times 0.5 = 12.5 $
- The standard deviation will be $\sqrt{\sigma^2} = \sqrt{12.5}$
- The value 35 and 15 are 10 away from the average, which is 2.8284 standard deviations (ie, $10 / \sqrt{12.5}$)
Using Chebyshev's Inequality we can write the following probability:
$$Pr( X <15 \cup X>35) = Pr(|X - \mu| \geq k \times \sigma) \leq \frac{1}{k^2} = \frac{1}{2.8284^2} = 0.125$$
In other words, chances of a fair coin coming up heads outside the range of 15 to 35 times is at most 0.125.
Python Implementation
We'll first import a few libraries that will help us work with Chebyshev:
Chebyshev's inequality can be written as a simple function:
We'll now use this function to find probability for different k values. First, we'll create an array of 10 numbers that have a distance of 0.5 between values. Letβs also initialize an array of zeros of the same length in order to hold the probability results weβre about to calculate.
For comparison, find the probability for unit normal distiribution, which we'll plot in the next step:
Now, we'll plot the probability for Normal Distribution and Chebyshev Inequality:
The figure shows that Chebyshev's Inequality provides an upper bound (the blue curve) for the true ratio of large numbers that can be drawn from a unit normal distribution (the orange curve). Note that Chebyshevs's Inequality provides tighter bounds for larger k values.
A Python Example
We will load the penguins
dataset from the seaborn
library to observe Chebyshev's Inequality. In the dataset, the flipper_length_mm
variable will be used to estimate an interval using the k
parameter.
First, we'll plot the distribution of flipper length:
The figure shows that the the variable does not follow a normal curve. Nonetheless, we can still use Chesbyshev's Inequality for estimation.
Let's now calculate the mean and standard deviation for our calculations:
Using the mean, standard deviation, and the k parameter, we can now find the upper and lower bound:
We can obtain a kernel density function using the KernelDensity
class from the sklearn library:
Now, let's plot the density model:
Here, the red lines indicate lower-bound and upper-bound for k=2. The green vertical line shows the mean of the data. The filled area under the curve shows the values between $\mu-2\sigma$ and $\mu-2\sigma$.
Using the results, we can easily detect outliers. The values higher than upper-bound and the values less than lower-bound can be flagged as outliers.
Here's how to find the values less than lower_bound
:
And the values higher than upper_bound
: