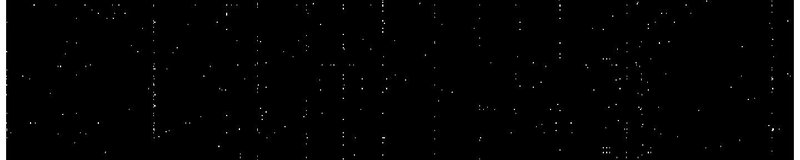
Sparse Matrix
LearnDataSci is reader-supported. When you purchase through links on our site, earned commissions help support our team of writers, researchers, and designers at no extra cost to you.
What is a sparse matrix?
A sparse matrix is a special case of a matrix in which the number of zero elements is much higher than the number of non-zero elements. As a rule of thumb, if 2/3 of the total elements in a matrix are zeros, it can be called a sparse matrix. Using a sparse matrix representation — where only the non-zero values are stored — the space used for representing data and the time for scanning the matrix are reduced significantly.
Many applications in data science and machine learning involve sparse matrices, such as:
- Natural Language Processing: The occurrence of words in documents can be represented in a sparse matrix. The words in a document are only a small subset of words in a language. If we have a row for every document and a column for every word, then storing the number of word occurrences in a document has a high percentage of zeros in every column.
- Recommendation Systems: A sparse matrix can be employed to represent whether any particular user has watched any movie. See our Locality Sensitive Hashing (LSH) article for an example.
- Market Basket Analysis: Since the number of purchased items is tiny compared to the number of non-purchased items, a sparse matrix is used to represent all products and customers.
Numerical example 1
Let's take the example of a movie recommendation system. There are millions of users and thousands of movies, so it's not possible for users to watch and rate all movies. This data can be represented as a matrix where the rows are the users, and the columns are the movies.
Most of the matrix elements will be empty, where the missing values will be replaced with zeros. Since a small percentage of the matrix has non-zero values, this matrix can be considered a sparse matrix. A small portion of the data is given below:
Movie1 | Movie2 | Movie3 | Movie4 | Movie5 | Movie6 | Movie7 | |
---|---|---|---|---|---|---|---|
User1 | 0 | 0 | 0 | 3 | 0 | 0 | 4 |
User2 | 0 | 5 | 0 | 0 | 0 | 0 | 0 |
User3 | 0 | 0 | 5 | 0 | 0 | 4 | 0 |
User4 | 4 | 0 | 0 | 0 | 0 | 0 | 1 |
User5 | 0 | 2 | 0 | 0 | 3 | 0 | 0 |
The sparsity of this matrix can be calculated by obtaining the ratio of zero elements to total elements. For this example, sparsity is calculated as:
$$\begin{align} sparsity &= \frac {n_{zeros}}{n_{total}} \\[.5em] &= \frac{26}{35} \\[.5em] &= 0.742 \end{align}$$
It can be seen that the number of zeros in a sparse matrix is very high. Representing all zero values in a matrix like this would result in high memory usage, so in practice, only non-zero values of the sparse matrix are stored.
Numerical example 2
Another example would be to use a matrix to represent the occurrence of words in documents. The term-document matrix dimension will be $n \times m$, where $n$ is the number of documents and $m$ is the number of words in the language model. As a result, most of the matrix elements will be zero since only non-zero values are important for data analysis. In addition to a large amount of space used, there will be a computational time problem because all elements will be scanned to access non-zero elements. This process yields a computational complexity problem.
To overcome these problems, we can use different data structures to represent a sparse matrix. One common representation format for a sparse matrix is a Coordinate list (COO), which uses three-element tuples to store non-zero values' coordinates in a matrix. For example, the following table can be constructed to represent a sparse term-document matrix:
Row | Column | Value |
---|---|---|
0 | 3 | 3 |
0 | 6 | 4 |
1 | 1 | 5 |
2 | 2 | 5 |
2 | 5 | 4 |
3 | 0 | 4 |
3 | 6 | 1 |
4 | 1 | 2 |
4 | 4 | 3 |
In this table, indices of rows and columns of non-zero values are stored in a sparse representation. Let $k$ be the number of non-zero elements in a matrix of size $n \times m$, then the proportion of the space saved by sparse matrix representation can simply be calculated as follows:
$$ p = 1- \frac{3k}{nm} $$
The space gained by a sparse matrix representation is directly proportional to the sparsity value.
There are many other ways to represent a sparse matrix, such as Dictionary of keys (DOK) and List of lists (LIL). In the following section, different representation formats will be explained with Python.
Sparse Matrix in Python
The Scipy library provides the scipy.sparse
package to create and manipulate sparse matrix (https://docs.scipy.org/doc/scipy/reference/sparse.html. Different representation formats and some useful functions for sparse matrices are defined in this package.
Here we will explore some basic functions.
A simple, sparse matrix
A simple, sparse matrix will be constructed to show the representation formats of a sparse matrix in Python.
There's many zeros in this matrix, so let's calculate the sparsity of the matrix:
We can convert this dense matrix into a sparse matrix by using the sparse.csr_matrix()
function. The row/column indices of non-zero values are stored in a Compressed Sparse Row (CSR) matrix:
Another efficient structure for constructing sparse matrices is the Dictionary Of Keys (DOK), where a python dictionary is used to represent non-zero values for a sparse matrix.
In this representation, keys()
is used for indices, and values()
is used for values of non-zero elements:
The last representation format shown here is a row-based list of lists sparse (LIL) matrix. The first list stores column indices for each row, and the second list is used to store the element's row values.
In scipy.sparse
package, there is also a todense()
function for converting a sparse matrix to a dense matrix:
This can be useful when exploring data, but if your dataset is large, the dense matrix version won't fit in memory and may cause an error.
Sparse Matrix from a real dataset
We'll use the newsgroups dataset, available directly from sklearn, to show an example of when a sparse matrix would be used. This dataset contains thousands of news posts on 20 different topics.
A document can be represented as a term vector, where each term is a feature and the value is the number of times the corresponding term occurs in the document. In this way, a matrix can be used to represent word occurrences in all documents, where the documents are the rows and the terms are the columns. Since the number of non-zero values will be small, this matrix can be represented as a sparse matrix.
Let's import and load the dataset from sklearn:
Previewing an example entry in this dataset:
Now we'll use CountVectorizer
to vectorize the text into a term-document matrix:
Next, we'll can calculate the sparsity:
Convert the sparse matrix to a dense matrix:
With the dense version we can calculate the proportion of space saved by sparse matrix represantation: